Berkeley Disaster Lab Academics Mobilize AI Solutions to Confront Climate Change Disruptions.
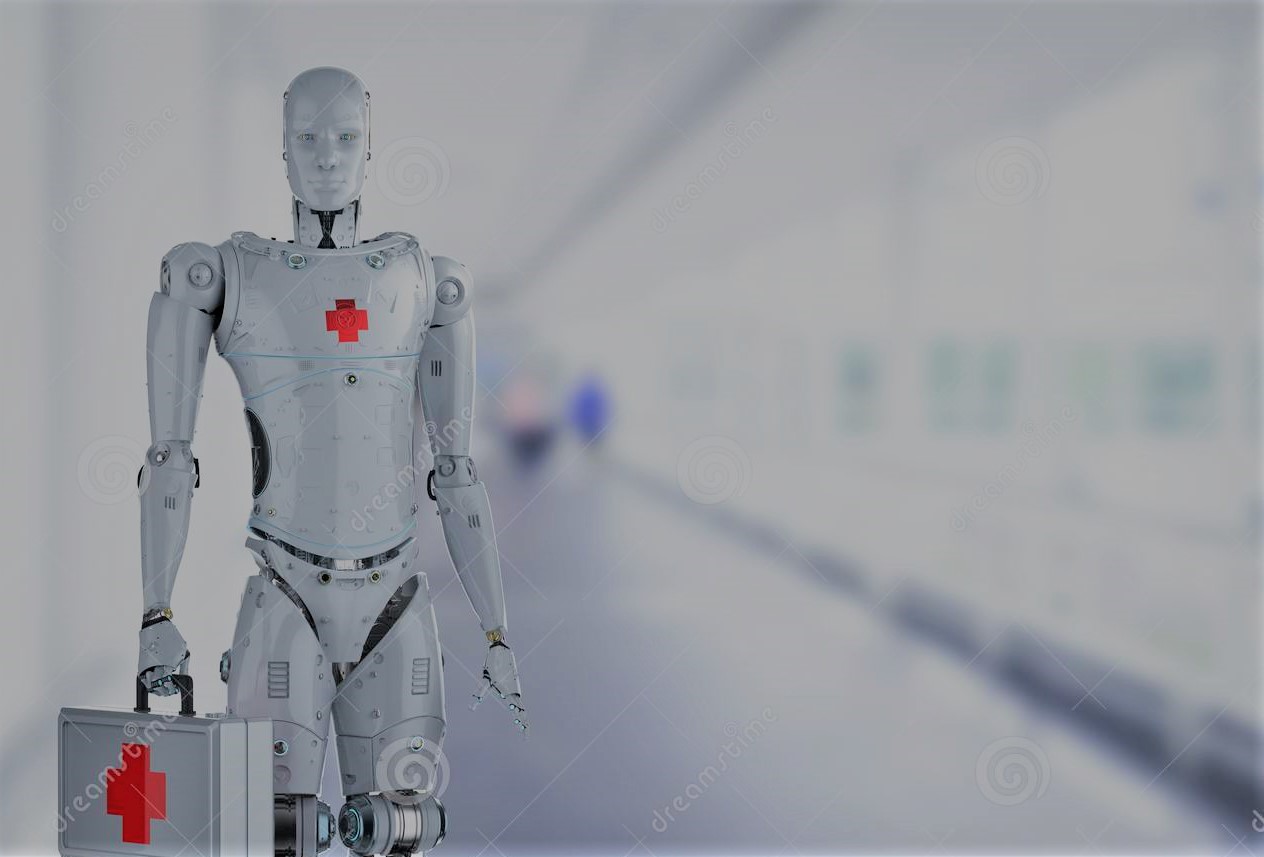
“Sixty percent of the twenty-five countries most affected by climate change are affected by conflicts and wars. Climate hazard adaptation is lacking in the humanitarian landscape*.” Patrick Youssef, Regional Director, Africa, International Committee of the Red Cross (ICRC).
Navigating uncertainty and ambiguity.
Humanitarian Assistance is facing new pressures and disruptions from the triple Covid-19, climate change, and conflict paradigm. Reliable quality of life data is crucial for humanitarian organizations to develop targeted interventions for vulnerable communities, especially in times of crisis. New tools and mechanisms are needed to better mitigate and resolve this challenge. Artificial Intelligence (AI) practitioners are optimizing and tuning AI solutions to deliver the best possible real-time performance for Humanitarian Assistance and Disaster Relief (HADR) practitioners. “How to build robots that can crawl around the rubble and find people. How can we stop the spread of misinformation on social media during and after a disaster, which is common? How do we understand disaster forecasting? If there are floods, how do we forecast where the high-water mark will be? How do we forecast what will be impacted? What critical infrastructure is impacted?” With these challenges in mind, AI engineers are developing compelling and meaningful proposals for products, services, and systems to provide fast and reliable data. Concrete initiatives and actions are in the work. In December 2021, UC Berkeley undergraduate and graduate students at the Disaster Lab[1]s X-Lab Innovation facilitated The AI + HADR Workshop[2] “to consider new insights, data, and prototypes solutions to key problems identified by first responders”. Ritwik Gupta, the event’s chair and a machine learning research scientist at Carnegie Mellon University‘s Software Engineering Institute (SEI)’s Emerging Technology Center (ETC), and Eric Heim, a senior machine learning research scientist at the ETC, were the primary organizers. For Gupta, this effort[3] is “to spur innovation in the disaster technology space in regard to home hardening, defensible space, early detection, and rapid response” among researchers and industry experts.
Ecological Threat Register 2020 Key Findings.
“The Ecological Threat Register (ETR)[4] analyses risk from population growth, water stress, food insecurity, droughts, floods, cyclones, rising temperatures, and sea levels. The ETR analyses the levels of societal resilience within countries to determine whether they have the necessary coping capacities to deal with future ecological shocks.
•19 countries with the highest number of ecological threats are among the world’s 40 least peaceful countries including Afghanistan, Syria, Iraq, Chad, India, and Pakistan.
• Over one billion people live in 31 countries where the country’s resilience is unlikely to sufficiently withstand the impact of ecological events by 2050, contributing to mass population displacement.
• Sub-Saharan Africa, South Asia, the Middle East, and North Africa are the regions facing the largest number of ecological threats.
• By 2040, a total of 5.4 billion people – more than half of the world’s projected population – will live in the 59 countries experiencing high or extreme water stress, including India and China.
• 3.5 billion people could suffer from food insecurity by 2050, which is an increase of 1.5 billion people from today.
• The lack of resilience in countries covered in the ETR will lead to worsening food insecurity and competition over resources, increasing civil unrest and mass displacement, exposing developed countries to increased influxes of refugees.”
Developing a neoteric social-technical fluency.
Despite increased weather-related world disaster events, the use of robots, AI, and ML in HADR scenarios remains in its infancy. Because of HADR’s dynamic and context-specific nature, disconnects between innovative technologies development and implementers on the ground prevail, along with data gaps and data ownership challenges. Working within interdisciplinary teams, Gupta would like to develop a neoteric social-technical fluency to better understand stakeholder needs; to provide non-technical data-sources tools and platforms; and, to communicate outcomes to first responders: “exploring methods focused on exploiting vision-based modalities to have downstream impacts in the world of humanitarian assistance and disaster response.”
The methodology is four-fold:
1/ Observe and Notice: connect courses and students with a network of stakeholders to bring in new voices onboard.
2/ Frame and Reframe: scoping actionable and accessible problems.
3/ Make and Experiment: create new tools, data, and assets to invite non-technical students to foster “real interdisciplinary hunger to engage”.
4/ Connections and platforms: help student teams to engage with first responders.
Phasing out digital literacy tensions.
Science, applied to real-world systems requires innovative processes and experiments with IT platforms, involving data transfer, data processing, and data interpretation. Digital literacy tensions are unavoidable from HADR’s diverse organizations and management processes involved. HADR multi-stakeholder practitioners must develop and cultivate a shared language to address users’ solutions, customers’ needs, and systems constraints. Technical bottlenecks, unique flow, data gaps, and unmet needs are a challenge for the implementation and effectiveness of AI solutions. For instance, the regulatory environment for drone usage can be a source of blockage to finding survivors. Non-technical issues such as funding can offset innovative solutions. Many historical useful instruments have been discontinued. Fortunately, although not to its full potential, the prototype device, FINDER (Finding Individuals for Disaster and Emergency Response) is being deployed some disaster theaters. However, a lack of funding or interest from the private sector has prevented its wider use. First evaluated in the aftermath of the 2010 Haiti earthquake, FINDER helped search and rescue teams to save the lives of four people using radar waves to detect the heartbeats of survivors trapped in collapsed buildings after the 2015 Nepal earthquake.
Conclusion:
Understanding the complex linkage between the social and the technical.
The outcome of the workshop demonstrated the advance of AI practitioners in applying statistical machine learning and AI capabilities into the HADR’s different geography and dynamic nature-specific context. Developing insights by listening, Gupta and his team can reframe and transfer AI knowledge into user-friendly applications and insights to first responders and emergency planners. Leveraging disparate data sets while engaging content experts, from first responders to academics, the HADR community has developed a neoteric social-technical fluency to drive change and save more lives.
The following projects were among those submitted to the AI for Humanitarian Assistance and Disaster Response (AI+HADR) workshop, NeurIPS 2021.
Climate Change Preparedness
Mapping Access to Water and Sanitation in Colombia using Publicly Accessible Satellite Imagery, Crowd-sourced Geospatial Information and Random Forests.
Quality-of-life data is access to water, sanitation and hygiene (WASH). Traditionally, data collection is done through door-to-door surveys sampled over large areas. A combination of machine learning and census data, publicly available satellite images, and crowd-sourced geospatial information generated a map of WASH able to explain up to 65% of the variation in predicting access to water supply, sewage, and toilets.
2111.04134v1.pdf (arxiv.org)
On pseudo-absence generation and machine learning for locust breeding ground prediction in Africa.
Desert locust outbreaks threaten the food security of a large part of Africa and have affected the livelihoods of millions of people over the years. Machine learning (ML) logistic regression combined with more advanced pseudo-absence generation, specifically environmental profiling, can be a sensible and effective approach to predicting locust breeding grounds across Africa.
2111.03904.pdf (arxiv.org)
Deep Learning Methods for Daily Wildfire Danger Forecasting
Wildfire forecasting is of paramount importance for disaster risk reduction and environmental sustainability. Daily fire danger prediction as a machine learning task uses a set of covariates that jointly affect the fire occurrence and spread, such as weather conditions, satellite-derived products, topography features and variables related to human activity. Deep Learning (DL) models provide national-scale daily fire danger maps at a much higher spatial resolution than existing operational solutions.
2111.02736.pdf (arxiv.org)
Infrastructure damage mitigation
Building Damage Mapping with Self-Positive Unlabeled Learning
Deep learning approaches are difficult to implement in real-world disasters because it might be challenging to collect ground truth data of the damage situation (training data) soon after the event. The implementation of recent self-paced positive-unlabeled learning (PU) is demonstrated in this work by successfully applying to building damage assessment with limited labeled data and a large amount of unlabeled data.
2111.02586.pdf (arxiv.org)
Unsupervised Change Detection of Extreme Events Using ML On-Board
Applications such as disaster management enormously benefit from the rapid availability of satellite observations. Traditionally, data analysis is performed on the ground after all data is transferred – downlinked – to a ground station. RaVAEn pre-processes the sampled data directly on the satellite and flags changed areas to prioritise for downlink, shortening the response time. RaVAEn outperforms pixel-wise baselines.
2111.02995.pdf (arxiv.org)
Damage Estimation and Localization from Sparse Aerial Imagery
Aerial images provide important situational awareness for responding to natural disasters such as hurricanes. They are well-suited for providing information for damage estimation and localization (DEL); i.e., characterizing the type and spatial extent of damage following a disaster. The performance on post-event data from the 2016 Louisiana floods achieved a precision of 88%. Given this high precision using limited data, this approach is currently viable for fast and effective DEL from handheld aerial imagery for disaster response.
2111.03708v2.pdf (arxiv.org)